Likelihood Estimation of Secondary Crashes Using Bayesian Complementary Log-Log Model
Document Type
Article
Publication Date
10-1-2018
Publication Title
Accident Analysis and Prevention
Abstract
© 2018 Secondary crashes (SCs) occur within the spatial and temporal impact range of a primary incident. They are non-recurring events and are major contributors to increased traffic delay, and reduced safety, particularly in urban areas. However, the limited knowledge on the nature of SCs has largely impeded their mitigation strategies. The primary objective of this study was to develop a reliable SC risk prediction model using real-time traffic flow conditions. The study data were collected on a 35-mile I-95 freeway section for three years in Jacksonville, Florida. SCs were identified based on travel speed data archived by the Bluetooth detectors. Bayesian random effect complementary log-log model was used to link the probability of SCs with real-time traffic flow characteristics, primary incident characteristics, environmental conditions, and geometric characteristics. Random forests technique was used to select the important variables. The results indicated that the following variables significantly affect the likelihood of SCs: average occupancy, incident severity, percent of lanes closed, incident type, incident clearance duration, incident impact duration, and incident occurrence time. The study results have the potential to proactively prevent SCs.
Recommended Citation
Kitali, Angela E.; Alluri, Priyanka; Sando, Thobias; Haule, Henrick; Kidando, Emmanuel; and Lentz, Richard, "Likelihood Estimation of Secondary Crashes Using Bayesian Complementary Log-Log Model" (2018). Civil and Environmental Engineering Faculty Publications. 255.
https://engagedscholarship.csuohio.edu/encee_facpub/255
DOI
10.1016/j.aap.2018.07.003
Volume
119
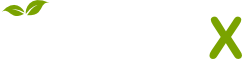
- Citations
- Citation Indexes: 45
- Usage
- Abstract Views: 2
- Captures
- Readers: 76
- Mentions
- News Mentions: 1