An End-to-End Network for Co-saliency Detection in One Single Image
Document Type
Article
Publication Date
11-2023
Publication Title
Science China: Information Sciences
Abstract
Co-saliency detection within a single image is a common vision problem that has not yet been well addressed. Existing methods often used a bottom-up strategy to infer co-saliency in an image in which salient regions are firstly detected using visual primitives such as color and shape and then grouped and merged into a co-saliency map. However, co-saliency is intrinsically perceived complexly with bottom-up and top-down strategies combined in human vision. To address this problem, this study proposes a novel end-to-end trainable network comprising a backbone net and two branch nets. The backbone net uses ground-truth masks as top-down guidance for saliency prediction, whereas the two branch nets construct triplet proposals for regional feature mapping and clustering, which drives the network to be bottom-up sensitive to co-salient regions. We construct a new dataset of 2019 natural images with co-saliency in each image to evaluate the proposed method. Experimental results show that the proposed method achieves state-of-the-art accuracy with a running speed of 28 fps.
Repository Citation
Yue, Yuanhao; Zou, Qin; Yu, Hongkai; Wang, Qian; Wang, Zhongyuan; and Wang, Song, "An End-to-End Network for Co-saliency Detection in One Single Image" (2023). Electrical and Computer Engineering Faculty Publications. 517.
https://engagedscholarship.csuohio.edu/enece_facpub/517
DOI
10.1007/s11432-022-3686-1
Volume
66
Issue
11
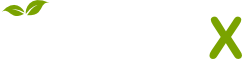
- Citations
- Citation Indexes: 6
- Usage
- Abstract Views: 14
- Captures
- Readers: 15
- Mentions
- News Mentions: 1
Comments
This work was supported by Key Research and Development Program of Hubei Province (Grant No. 2020BAB018), National Natural Science Foundation of China (Grant No. 62171324), and National Key R&D Program of China (Grant No. 2022YFF0901902).