Heterogeneous Trajectory Forecasting via Risk and Scene Graph Learning
Document Type
Article
Publication Date
11-2023
Publication Title
IEEE Transactions on Intelligent Transportation Systems
Abstract
Heterogeneous trajectory forecasting is critical for intelligent transportation systems, while it is challenging because of the difficulty for modeling the complex interaction relations among the heterogeneous road agents as well as their agent-environment constraint. In this work, we propose a risk and scene graph learning method for trajectory forecasting of heterogeneous road agents, which consists of a Heterogeneous Risk Graph (HRG) and a Hierarchical Scene Graph (HSG) from the aspects of agent category and their movable semantic regions. HRG groups each kind of road agents and calculates their interaction adjacency matrix based on an effective collision risk metric. HSG of driving scene is modeled by inferring the relationship between road agents and road semantic layout aligned by the road scene grammar. Based on this formulation, we can obtain an effective trajectory forecasting in driving situations, and superior performance to other state-of-the-art approaches is demonstrated by exhaustive experiments on the nuScenes, ApolloScape, and Argoverse datasets.
Repository Citation
Fang, Jianwu; Zhu, Chen; Zhang, Pu; Xue, Jianru; and Yu, Hongkai, "Heterogeneous Trajectory Forecasting via Risk and Scene Graph Learning" (2023). Electrical and Computer Engineering Faculty Publications. 525.
https://engagedscholarship.csuohio.edu/enece_facpub/525
DOI
10.1109/TITS.2023.3287186
Volume
24
Issue
11
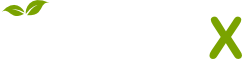
- Citations
- Citation Indexes: 9
- Usage
- Abstract Views: 1
- Captures
- Readers: 17
- Mentions
- News Mentions: 1